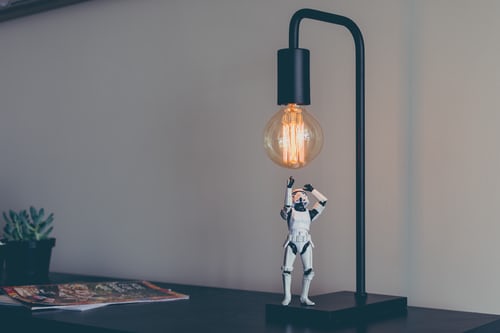
Sixty years ago this week, scientists at Georgetown and IBM lauded their machine translation «brain,» known as the 701 computer. The «brain» had successfully translated multiple sentences from Russian into English, leading the researchers to confidently claim that translation would be fully handled by machines in «the next few years.»
Fast forward six decades, and MIT Technology Review makes a remarkably similar proclamation: «To translate one language into another, find the linear transformation that maps one to the other. Simple, say a team of Google engineers.»
Simple? Not exactly.
Even in the 1950s, IBM acknowledged that to translate just one segment «necessitates two and a half times as many instructions to the computer as are required to simulate the flight of a guided missile.» It’s also highly doubtful that the scientists at Google see anything «simple» about their new method, which relies on vector space mathematics.
Granted, there is a beautiful simplicity in statistical machine translation, such as Google Translate. Essentially, the more data you have, the better the probability of a high-quality translation as an end result. But what do you do when you don’t have enough data? Or in the case of Google, what do you do when the data might be out there somewhere, but it isn’t part of the free and public web that you’re designed to mine?
That’s when you come up with new techniques, just as Google has done. Their new method — one that is meant to complement, but not replace their statistical approach — automatically creates dictionaries and phrase tables without help from humans. The new technique uses data mining in order to compare the structure of one language to another, and then generates phrase tables and dictionaries accordingly. This means that Google won’t have to rely exclusively on documents available in two languages to improve its translation quality. It will have other methods, such as this new one, to add to the mix.
What does this mean? Even Google isn’t satisfied that statistical machine translation will move things along quickly enough. That method has its limitations, just like all methods do.
What’s fascinating is that every few months, starry-eyed and often misinformed journalists herald a new era for language translation, announcing a «groundbreaking milestone» related to a technology that has been around for 60 years.
And their claim is always the same: «The translation problem is solved!»
Unfortunately, equating such minor machine translation accomplishments with «solving the translation problem» is like assuming that because we’ve walked on the moon that we can all just pack up and move there. We can’t, and we may never be able to. But that doesn’t stop us from trying.
Machine translation, or computer-generated translation as we often call it at Smartling, is a technical marvel. It serves many important purposes, can be used properly in specific, limited cases, and is useful for a variety of tasks that are typically unrelated to the final output on the page. Personally, I’m a believer in making strides toward improving machine translation. For that reason, I profiled the work of Franz Och, the brain behind Google Translate, in Found in Translation.
However, machine translation is not going to replace professional human translators anytime soon. Here are six reasons why:
1. It’s Tough to Get Good Translation, Even From Perfectly Bilingual Human Beings.
One of the reasons that machine translation cannot replace professional human translation is the same reason that plain old bilingual laypeople, for many tasks, cannot replace professional human translation. For most translation jobs, the task of translation requires more than just knowledge of two languages. The idea that you can simply create one-to-one equivalencies across languages is false.Translators are not walking dictionaries. They recreate language. They craft beautiful phrases and sentences to make them have the same impact as the source. Often, they devise brand-new ways of saying things, and to do so, they draw upon a lifetime’s worth of knowledge derived from living in two cultures. Machines cannot exactly do that.
2. Translation Quality is Highly Subjective.
Even if machines could approximate human translation quality, it’s unclear which version of human quality they would emulate. Give a text to 100 human translators, and you’ll get 100 different translations. Which one offers the best «quality?» In many ways, this is like asking someone which rendition of a song is best when sung by 100 different singers. Your choice will be subjective in many ways, even if you can argue that one artist hit a flat note while another had perfect pitch. While this diversity of human language expression makes things complicated, it’s also a necessity. Machine translation tools, so far, present far more limited options with their output, which are generally too simplistic for the complex linguistic realities of most translation projects.
3. There Are Too Many Languages Out There.
Google Translate today supports 80 languages. There are between 6,000 and 7,000 languages alive today, of which about 2,000 are considered endangered. If we use a very conservative estimate and say there are only 1,000 languages of significant economic importance in the world today, that still leaves 920 languages yet to be developed. If Google were to add 10 languages per year, it would take 92 years for us to see even a fraction of the world’s human languages addressed through machine translation. Most of us won’t be around by then, meaning that machine translation — even at the poorest levels of quality — will not be a reality for the majority of the world’s languages during our lifetime.
4. Most Languages Are Not Written.
The vast majority of the world’s languages are spoken or signed. Online, much of our communication is migrating from text to a combination of text plus audio, and even more importantly, video, which encompasses audio as well and helps us leap past text. This means that written language need not be the barrier it once was for people whose languages lack written forms. It also means that translation has its limits. Spoken language suddenly takes on new importance as the internet travels to places far-flung. Smartphones and tablets with visual, tactile and audio inputs make text less important in our world. This doesn’t mean that text translation won’t be important. It might just mean it will increasingly take place behind the scenes, with audio or video output instead.
5. Context Ss Key.
In a language like English, a single word can have hundreds of different meanings, depending on the context (see «Clear Examples of Why Context Matters»). In fact, the Oxford English Dictionary’s lexicographer for the letter «R,» Peter Gilliver, claimed that the verb-form alone of «run» has no less than 645 distinct meanings. Can a machine learn each of these meanings for every word in not just one language, but two? This isn’t an easy question to answer. In fact, the OED explains that even the very nature of what constitutes a word is up for debate:
«It’s impossible to count the number of words in a language, because it’s so hard to decide what actually counts as a word. Is dog one word, or two (a noun meaning «a kind of animal,» and a verb meaning «to follow persistently»)? If we count it as two, then do we count inflections separately too (e.g. dogs = plural noun, dogs = present tense of the verb). Is dog-tired a word, or just two other words joined together? Is hot dog really two words, since it might also be written as hot-dog or even hotdog?»
Not only that, but word-for-word translation is impossible, so instead of thinking about words, when humans use context to figure out meaning, we think not just of single words, but how those words interact with the ones around them. Those combinations are constantly changing and multiplying, limited only by human creativity. Machines can hardly keep up.
6. Language Is Simply Too Important.
How important are the words your company uses to describe its products or services? They are critical. For many companies — including, perhaps ironically, Google — the voice of the brand all centers around word choice. How human beings make choices about the products they buy and the services they use relates directly to the words that are used to market and sell them. Perhaps when machines are the ones doing the buying, they’ll be less picky about language. For now, humans are still the ones opening their wallets, and humans are a strange bunch, with very real and emotional reactions to language. Our taste or distaste for a particular term often relates to our upbringing, our culture and even our past experiences. Humans cannot accurately predict which words will annoy or confuse even the people we know best. How can we expect a machine to fare any better?
So, if machine translation can’t fix all our language woes anytime soon, why does the world keep celebrating each and every related milestone as if it were a major achievement? Well, because it really would be nice if cross-language issues could be simplified. In fact, humans would love it if communication matters in general — even monolingual ones — were less complex.
The bottom line is this: Computers will never fully solve the translation problem, and even to make micro-strides toward that audacious goal, they will need significant help from humans. The question isn’t, «Will we get there?» but rather, «How far will we get, and how fast?» In the meantime, the utopia of computer-generated translation is a dream worth having, albeit a recurring one.
Dejar una contestacion
Lo siento, debes estar conectado para publicar un comentario.